Generative Ai Landscape
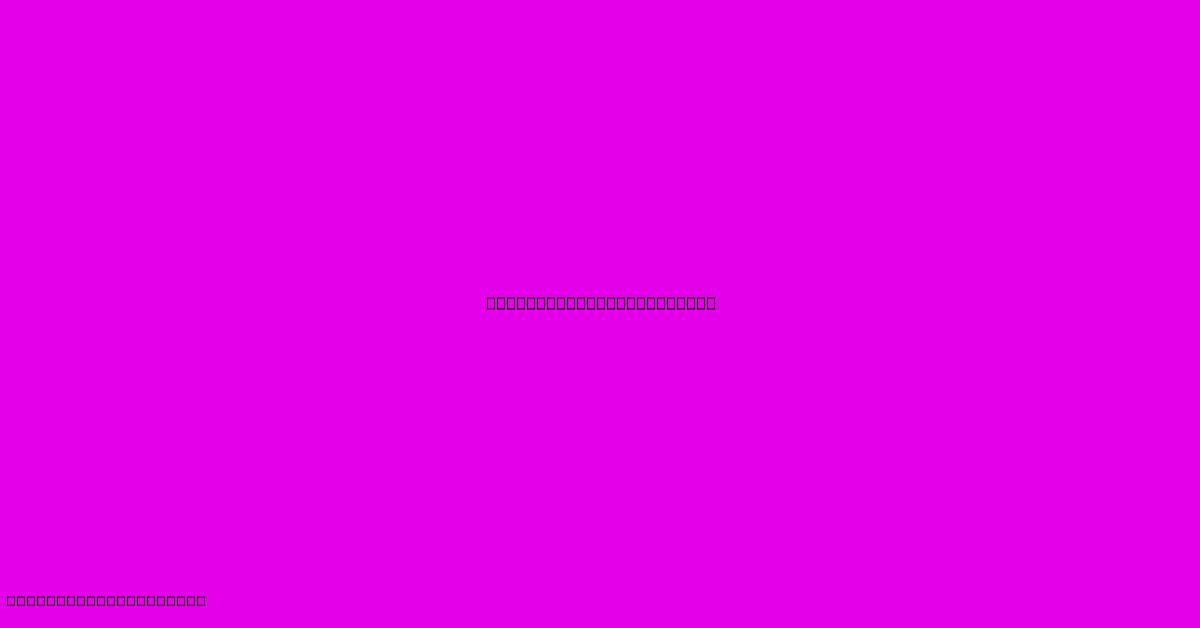
Table of Contents
Navigating the Generative AI Landscape: A Comprehensive Guide
The generative AI landscape is rapidly evolving, presenting both incredible opportunities and significant challenges. This comprehensive guide will explore the key players, technologies, applications, and ethical considerations shaping this transformative field. We'll delve into the nuances of this exciting space, providing you with a clear understanding of its current state and future potential.
Key Players in the Generative AI Arena
The generative AI space is a vibrant ecosystem with numerous players vying for dominance. These range from established tech giants to innovative startups, each contributing to the rapid advancement of this technology.
The Tech Giants:
- Google: A major force with models like PaLM 2, powering Bard and integrated into various Google services. Google's vast data resources and research capabilities solidify its position as a leader.
- Meta (Facebook): Actively developing generative AI models for various applications, including text, image, and video generation. Their focus on large language models (LLMs) and open-source initiatives is noteworthy.
- Microsoft: A significant investor in OpenAI, leveraging models like GPT-4 to enhance its products, including Bing Chat and GitHub Copilot. This partnership significantly impacts the competitive landscape.
- Amazon: Developing and deploying generative AI across its various services, including AWS, offering powerful tools and infrastructure for developers and businesses.
The Emerging Startups:
Numerous startups are pushing the boundaries of generative AI, focusing on niche applications and innovative approaches. These companies often specialize in specific areas, such as:
- Image generation: Companies like Midjourney and Stable Diffusion are leading the charge in creating stunning and realistic images from text prompts.
- Text-to-speech: Startups are developing advanced text-to-speech technologies with enhanced naturalness and expressiveness.
- Video generation: The field is still evolving, but startups are making significant strides in generating high-quality videos from text or other inputs.
Core Technologies Driving Generative AI
Several core technologies underpin the advancements in generative AI:
- Large Language Models (LLMs): These models, trained on massive datasets, are the foundation for many generative AI applications. They excel at generating text, translating languages, and answering questions. Examples include GPT-4, PaLM 2, and LaMDA.
- Generative Adversarial Networks (GANs): GANs consist of two neural networks competing against each other to generate realistic data, often used for image and video generation.
- Diffusion Models: These models gradually add noise to an image and then learn to reverse the process, generating high-quality images from noise. Stable Diffusion is a prime example.
- Transformer Networks: These architectures are crucial for processing sequential data like text and have revolutionized natural language processing (NLP).
Applications of Generative AI: A Wide-Ranging Impact
Generative AI's applications span numerous industries and sectors:
- Creative Industries: Generating art, music, literature, and marketing materials.
- Software Development: Assisting with code generation, documentation, and testing.
- Healthcare: Drug discovery, personalized medicine, and medical image analysis.
- Education: Personalized learning experiences and content creation.
- Customer Service: Developing chatbots and virtual assistants.
Ethical Considerations and Challenges
The rapid advancement of generative AI brings forth crucial ethical considerations:
- Bias and Fairness: Generative models can inherit and amplify biases present in their training data.
- Misinformation and Deepfakes: The potential for creating realistic but false content poses significant risks.
- Job Displacement: Automation driven by generative AI may lead to job losses in certain sectors.
- Intellectual Property Rights: Questions surrounding ownership and copyright of AI-generated content need careful consideration.
The Future of the Generative AI Landscape
The generative AI landscape is poised for continued rapid growth and innovation. We can expect:
- Improved model efficiency: Models will become more efficient, requiring less computational power and data.
- Enhanced creativity and control: Users will have more control over the creative process and output.
- Wider accessibility: Generative AI tools will become more accessible to a wider range of users.
- Increased regulation: Governments and organizations will likely implement regulations to address ethical concerns.
Navigating this evolving landscape requires a keen understanding of the technologies, applications, and ethical implications involved. By staying informed and engaging in responsible innovation, we can harness the transformative power of generative AI for the benefit of society.
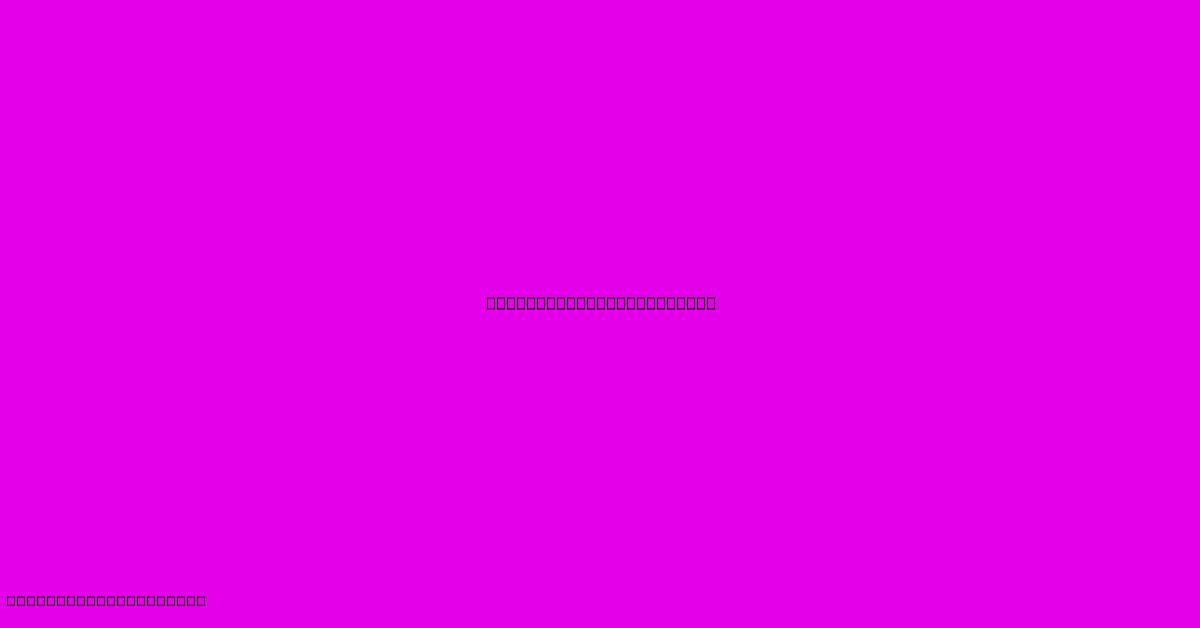
Thank you for visiting our website wich cover about Generative Ai Landscape. We hope the information provided has been useful to you. Feel free to contact us if you have any questions or need further assistance. See you next time and dont miss to bookmark.
Featured Posts
-
Gas Fireplace Insert Remote
Feb 02, 2025
-
Energy Saving Electric Fireplace
Feb 02, 2025
-
Ufc Saudi Arabia Israel Adesanya Ko D
Feb 02, 2025
-
Item In The Bathroom That Starts With E
Feb 02, 2025
-
Palm Beach Patio Set
Feb 02, 2025