Meta's Bias Solution: User Content Moderation
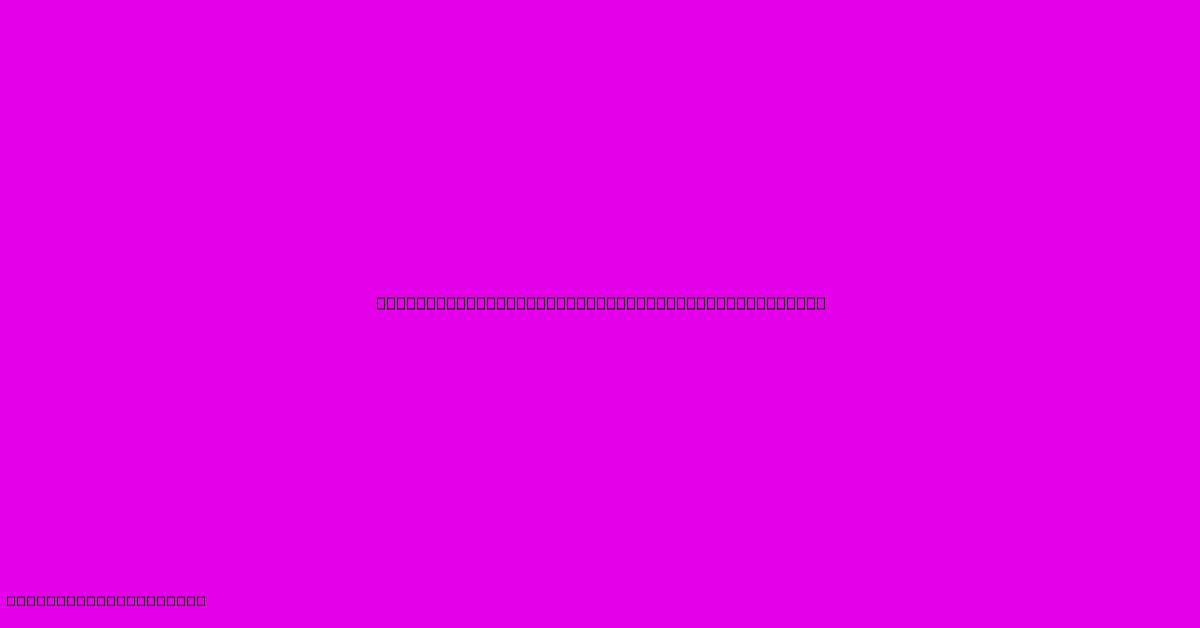
Table of Contents
Meta's Bias Solution: User Content Moderation – A Complex Balancing Act
Meta, formerly Facebook, faces a constant challenge: balancing free speech with the need to moderate user-generated content and prevent the spread of harmful information. This balancing act is further complicated by the inherent biases that can creep into both the algorithms and the human moderators tasked with enforcing content policies. This article delves into Meta's approach to addressing bias in user content moderation, exploring its complexities and limitations.
The Problem of Bias in Content Moderation
Bias in content moderation can manifest in several ways:
-
Algorithmic Bias: The algorithms used to identify and flag potentially harmful content can inherit biases present in the training data. This can lead to disproportionate targeting of certain groups or viewpoints. For example, an algorithm trained on a dataset primarily reflecting one cultural perspective might unfairly flag content from other cultures as offensive.
-
Human Moderator Bias: Human moderators, despite training, are susceptible to their own unconscious biases. Factors like personal beliefs, cultural background, and even fatigue can influence their judgments, leading to inconsistent application of content policies.
-
Data Bias: The very data Meta uses to train its algorithms and inform its policies can reflect existing societal biases. If the data predominantly reflects the views of a particular demographic, the resulting systems will likely reflect those biases.
Meta's Strategies to Mitigate Bias
Meta acknowledges these challenges and has implemented several strategies to mitigate bias in its content moderation:
1. Algorithmic Improvements:
-
Improved Training Data: Meta is actively working to diversify its training datasets, incorporating content from a wider range of sources and perspectives. This aims to create algorithms less likely to exhibit bias towards specific groups.
-
Transparency and Explainability: Efforts are underway to make the algorithms more transparent and explainable, allowing for better understanding of how decisions are made and identifying potential biases more easily.
-
Bias Detection and Mitigation Techniques: Meta employs various technical methods to detect and mitigate bias in its algorithms, including fairness-aware machine learning techniques.
2. Human Moderator Training and Oversight:
-
Extensive Training: Moderators undergo comprehensive training to understand Meta's content policies and recognize potential biases in their own judgments.
-
Appeals Processes: Robust appeals processes allow users to challenge content moderation decisions, ensuring fairness and accountability.
-
Diversity and Inclusion Initiatives: Meta aims to create a diverse workforce of moderators to reduce the influence of any single cultural perspective.
3. Community Standards and Policy Development:
-
Community Input: Meta actively seeks input from its community to inform the development and evolution of its content policies, striving for inclusivity and fairness.
-
Regular Policy Reviews: Policies are regularly reviewed and updated to reflect evolving societal norms and address emerging challenges.
-
External Audits: Independent audits are conducted to assess the effectiveness of Meta's content moderation practices and identify areas for improvement.
The Ongoing Challenge
Despite these efforts, addressing bias in content moderation remains a significant ongoing challenge. The scale of user-generated content, the complexity of human language and cultural contexts, and the rapid evolution of online discourse all contribute to the difficulty of creating a perfectly unbiased system.
Conclusion: A Necessary Evolution
Meta's efforts to mitigate bias in user content moderation are crucial for maintaining a safe and inclusive online environment. While a completely bias-free system remains an aspirational goal, ongoing investment in algorithmic improvements, human moderator training, and community engagement is essential. The journey towards a more equitable and unbiased platform is a continuous process requiring constant vigilance and adaptation. The success of these efforts will be determined by Meta's ongoing commitment to transparency, accountability, and a genuine dedication to fairness for all users.
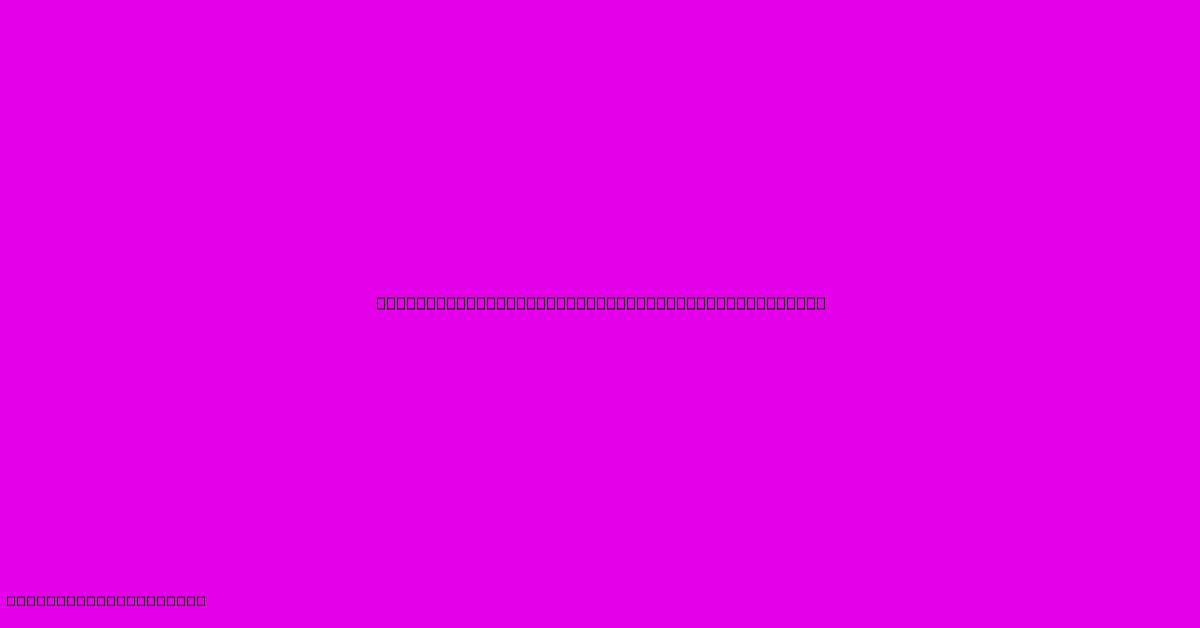
Thank you for visiting our website wich cover about Meta's Bias Solution: User Content Moderation. We hope the information provided has been useful to you. Feel free to contact us if you have any questions or need further assistance. See you next time and dont miss to bookmark.
Featured Posts
-
Nvidia Palantir Pull Dow Down
Jan 08, 2025
-
Spencer Heidis Home Fire Damage
Jan 08, 2025
-
Modern Red Dining Room
Jan 08, 2025
-
Value City 3 Piece Living Room Sets
Jan 08, 2025
-
Wittus Fireplace
Jan 08, 2025